Delivering the $1.25B/yr advertising platform for eBay sellers
Timeline
2017 - 2022 eBay Bellevue, WA
My deliverables
Created IA framework for Ads platform
Delivered Ads experience from blueprint to $1.25B
Grew PD team from 3 to 14 in four years
Platform Design Team
Operationally guided XFN team of 40
Metrics-centered design & delivery
Improved Design Intake process and drove output
Built effective XFN partnerships
Unified XFN team around the vision
My role
Lead UX Architect for Advertiser Experience
Product Design Manager for eBay Ads
Drove 0-1 delivery from sketch to launch
My team
eBay Ads
Ads program-level Product Managers
Ads-dedicated Engineering team
Design Team
User Research Team
Content Team
XFN
B2C Selling Product Managers
B2C platform Engineering team
Selling platform Design Team
Platform Design Team
User Research Team
Business Development Team
Legal Team
Goals

Aligning a large team around a new, enterprise-level initiative always presents a formidable challenge. The scale and complexity drive up the number of critically important steps before any work can commence. In the absence of shared and well-understood vision the development process, the resulting tolerances will stack into major misalignments, paving way to a colossal waste of time, resources and credibility.
Below are 6 key focus areas that I consider critical for a vision-level alignment.
Different roles, backgrounds and perspectives can only be an big asset when well-orchestrated.
Avoid overlaps in ownership areas. Assign responsibilities based on well-recognized qualifications.
Negotiating conflicting interests and priorities across XFN teams and stakeholders.
Ensure that all individual inputs are factual and rooted in data.
We perceive safety in what we know well. Balancing innovation with risk mitigation is crucial.
Reverse engineer the vision into smaller, measurable bits. Evaluate each bit on its own merit and risks.
The blank canvas effect can be overwhelming to many and allows for feelings to take over direction.
Seek factual data in prior relevant research, similar past initiatives and competitive studies.
Each of us has their own take on what we call “user-centric”, with small but widespread differences.
Position Research as defining source of truth for “user-centricity” based on qualitative evidence.
Not everyone is a great public speaker, but they may hold very valuable insights.
Empower each XFN member to contribute in an open and factual way. Be an active listener.
Turning goals into objectives

We needed to get into the next granular breakdown level by translating goals into objectives and, eventually, into JTBDs (informed by validated customer problems). Each breakdown point would exponentially tighten the precision of priorities, measurement, cost and risk mitigation.

Measurement

The project kick-off represents a collective surge of enthusiasm within the team. Energized by fresh challenges and the promise of new opportunities, multiple gears begin turning, propelling us toward action and creation. During this pivotal phase, precision becomes paramount as we align our definitions of success and failure. While initially committed to attributes like ‘user-centricity,’ ‘intuitiveness,’ and ‘clarity,’ we soon encounter diverse interpretations of seemingly common product qualities. Each team member develops their unique standard of ‘clarity,’ shaped by specific requirements and constraints and before long you may count as many definitions as there are members in the team.
This is why it is critical to collectively define the units which will be applied to calibrate the quality of the emerging experience at every agreed upon checkpoint.
Solving the right problems

Creating a successful and impactful product hinges on addressing the actual needs and pain points of the intended users. The resulting advantage is two-fold: it leads to a more resilient solution while fostering user satisfaction and loyalty from Day 1. Solving the right problem is the foundation of innovation and ensures that the product has a meaningful and enduring impact.
And this is where the role of User Research cannot be overstated. I was fortunate to have acquired a deep repository of seller and advertiser insights that had been accumulated over the previous 4 years. The bulk of those user pains laid dormant awaiting a framework-level solution.
I tasked myself with finding the intersection of user pains and the product objectives, all within the overall context and value of the horizontal seller toolset. Balancing familiarity with innovation also presented its special challenges.
Advertiser mental model

In the context of product design, mental models are often referred to as what a user believes about the system in hand. They are a set of conceptualized expectations that we all use to understand how something works. Their formation is a constant process that gradually evolves alongside us, through our use of each subsequent product. What we experience while interacting with products drives our expectation for the next interaction, and so on.
Advertising tools are no exception and (since the launch of Google AdWords in 2000) come with their own set of user expectations. Our goal was to find the balance between established systems and innovation, while grounding our decisions in the mental model of our sellers first.
Information architecture

The industry-defined objective of IA is to simplify the complexity. In complex systems design, the more befitting term is “turning complex into comprehensive”. The entire process of rationalizing multiple moving factors aims at answering one seemingly simple question: “Where can our users find the information they’re looking for?”. The investment in this stage pays off in a form of clear navigation, easy retrieval of relevant content and seamless scalability of features over time.
In this section I talk about adopting some of the celebrated IA principles towards specific outcome in a unique context of the Advertiser Experience.

User Interface

Advertising dashboard (L1)
Designing interfaces that handle large amounts of data presents unique challenges. These interfaces are prone to common pitfalls such as clutter and noise. Specifically, when it comes to dashboards—the central hub for generating insights—taking shortcuts can lead to a phenomenon known as “tolerance stacking.” This accumulation of small design compromises would ultimately impact advertising performance downstream.
Data-heavy interfaces are prone to common pitfalls such as clutter and noise. Specifically, when it comes to dashboards - the central hub for generating insights - taking shortcuts can lead to a phenomenon known as “tolerance stacking.” This accumulation of small design compromises would ultimately impact advertising performance downstream.
In the realm of data-driven interfaces, a strategic approach involves aggregating information into layers. These layers act as tiers, each revealing progressively more granular insights. The effectiveness of this approach hinges on the precise layering of reporting metrics. As sellers interact with the interface, information is disclosed gradually, aligning with their specific demands within each session.
By extensively incorporating qualitative data, the process of feature prioritization ensures that only essential information remains at the forefront of the user’s attention. The overall clarity of the interface cannot be solved by visual design alone. We needed to question every feature based on its user value, not merely on aesthetic trends.
The best product design does not aim at fulfilling the needs of every single user. Instead it aims at allowing users to adapt the interface towards their specific needs. With the virtually endless amount of user scenarios in front of us, investing heavily in customization was of strategic importance.
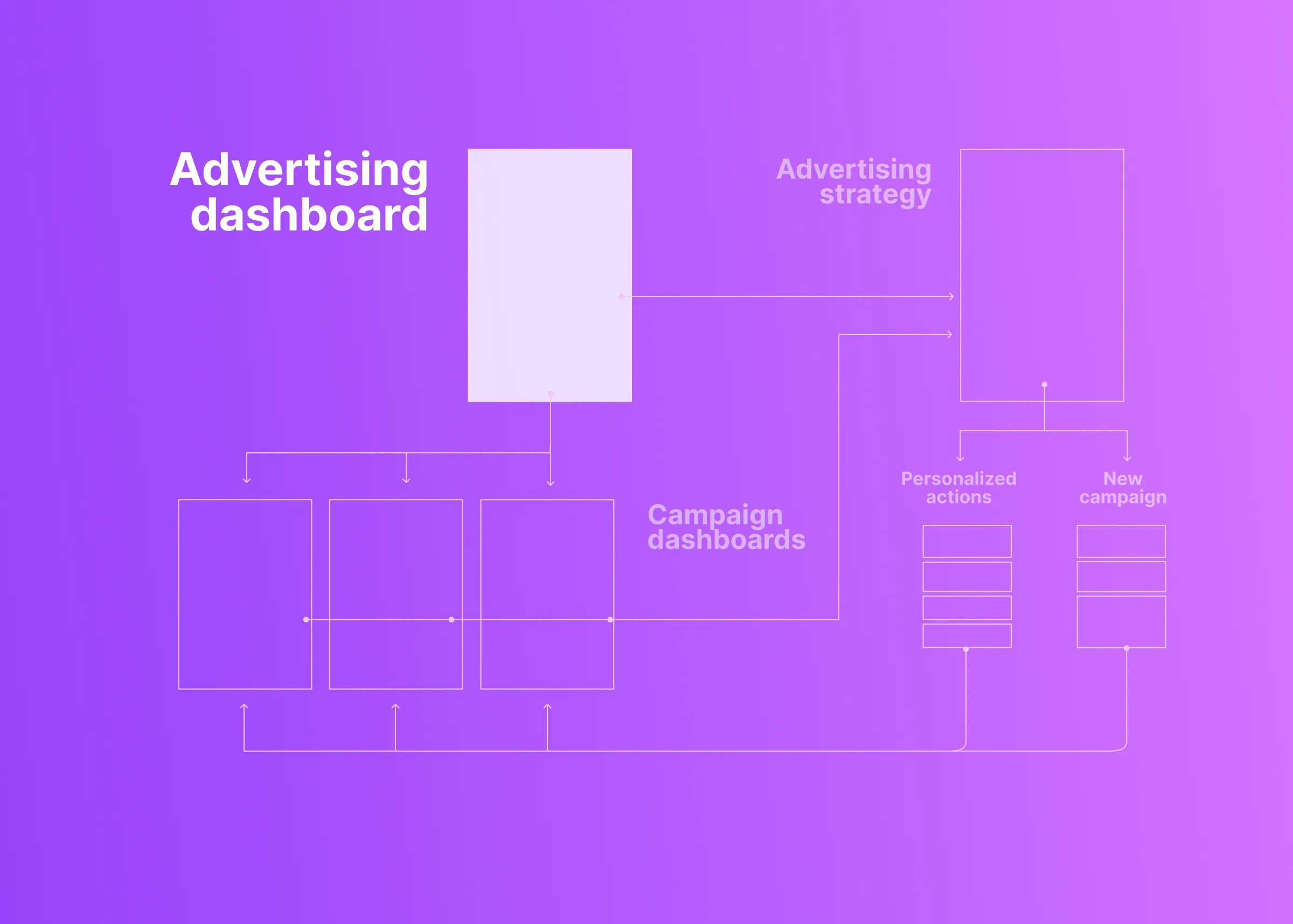


Campaign performance (L2 & L3)
While Advertising Dashboard provides the campaigns summary state, the Campaign Dashboard pages allow for more granular insights at the Ad Group, Listing and Keyword levels. The cumulative performance in the Dashboard does not reveal the over- and under-performance within each campaign. This is where a detailed L2 and L3 data helps to identify and make mid-flight performance adjustments.

Campaign Dashboard: Ad groups state (CPC)

Campaign creation flows
This was yet another section where the industry-standard signpost model required a design rethink. Instead of the common “step-by-step” creation flow I have designed and tested a consolidated “one-page” campaign creation that addressed a major seller ask - the ability to cross reference campaign parameters within a single surface. This was especially critical as we placed large bets on dynamic guidance for keywords and bid rates. Giving sellers the ability to easily triangulate all moving campaign parameters, without the intrusive pagination steps had become a fascinating design challenge.


The outcome

2,800% revenue growth (2017 - 2021)
By the middle of 2021, when all core framework components had been released, eBay was on its first ever trajectory to cross $1B in ads revenue for the reporting year. What’s even more notable, is the percentage-based share of Ads-driven revenue in annual earnings (0.45% vs 11.9%). This was proportional to Ads GMV gains as well as notable growth in seller adoption (more on this in the What We Missed section). I myself have attributed the significance of these gains to the careful balancing of seller-centric design with aggressive product objectives all along this unforgettable 4-year journey.
Ads contribution to total annual revenue at eBay:
2016
Total net revenue: $9,228M
Revenue share from Ads: 0.47%
2021
Total net revenue: $10,420M
Revenue share from Ads: 11.9%
We’ve earned trust and support
By transforming a significant concept into a major revenue stream, our Advertising platform has rightfully secured its position at the highest levels of strategic discussions. Our Leadership and investors have placed their trust in us, resulting in increased resources and greater autonomy to shape our long-term strategy. This trust has evolved into confidence, prompting us to allocate additional internal bandwidth for experimentation and embrace increasingly visionary initiatives. The momentum that would carry eBay Ads forward for many years had been set.
Smart use of resources
Rather than relying solely on a horizontal Design System, I’ve meticulously developed a set of universal Product Components to ensure the UX integrity of our Advertising Experience."This strategic implementation has yielded three pivotal advantages:
Across the suite of advertising programs, users encounter a consistent user interface (UI) and experience while performing identical tasks. This uniformity contributes to a decreased learning curve, which is particularly beneficial for smaller GMV segments.
The responsibilities for creating product components is thoughtfully distributed among development teams based on their unique skill sets. Once a component reaches a state of readiness, it is seamlessly integrated into a shared code-level library, accessible across all advertising programs.
The efficiencies gained through reduced development time have bestowed upon us an expanded opportunity to explore and validate hypotheses within controlled, staged environments. Consequently, this accelerated cycle has facilitated the rapid generation of valuable quantitative insights for eBay Ads development.
Improved XFN alignment and Design output
The design intake process plays a pivotal role in the success of any product organization. This is especially true in the context of building complex products and systems. I have successfully challenged the design delivery process, beginning with the most critical point - how designers intake design requests and how this affects the quality of resulting output.
Building a portfolio involves designing for multiple evolving products at once. This involves real-time partnerships with multiple stakeholders, which often brings on conflicting requirements. I have watched designers grapple with vague and conflicting directions fueled by product bias and aggressive timelines. The design organization was in dire need of expectations-setting alignment at the very beginning of the work streams, before initial confusion begins to impact output downstream.
The intake process encourages thorough scoping, allowing designers to focus on creative problem-solving rather than deciphering ambiguous requests. When expectations are managed proactively, frustration diminishes, and collaboration becomes more harmonious.
Repeat redesign efforts can be draining for design teams. The lingering misalignment is very corrosive to resulting output and to team morale. Designers get locked in a circle of endless re-think and fail to see tangible fruits of their labors. Creativity is replaced by labor-intensive churn and partnerships begin to fray, as design approach becomes reactive and increasingly short-sighted.
The intake process emphasizes clarity of design intent in alignment with business goals right from the starting line.
In the context of tightly woven product frameworks that involve contributions from multiple partners, designers found themselves navigating ad-hoc work sessions with individual product owners. During these alignment rounds, they encountered a barrage of conflicting opinions, each supported by distinct and often incompatible facts and data. Essentially, designers assumed a dual role: not only as creators of design solutions but also as program managers striving to harmonize platform-level objectives.
To address this challenge, we established a well-defined intake process. Rather than receiving inputs individually, XFN (cross-functional) partners were encouraged to participate collectively as a panel. By doing so, we facilitated real-time resolution of contradictions, leaving designers with clearly delineated and mutually agreed-upon objectives.
A supportive and fulfilled design team possesses a magnetic quality that not only attracts top talent but also entices existing designers to make lateral moves from other teams. Working culture becomes a leading incentive of itself and gives design leaders a robust selection of skill sets and healthy ambitions to put towards collective output.
The implementation of the regimented design process had contributed to the growth of design team over the years. Designers are able to see meaning in their work, then turn the fruits of their real impact into measurable career growth steps. Fostering a clear sense of purpose nourishes our creative egos in the most ethical way imaginable. Impactful design outcomes make it easy for a leader to recognize and reward the contributors, further perpetuating the nourishment cycle.
What we got wrong

Unintentional seller segment bias
Reflecting upon the journey toward finalizing the advertising experience, I’ve come to realize that we’ve inadvertently overlooked a seemingly insignificant truth. As our functionalities delved deeper, fueled by ever more granular data streams, the overall complexity of what was initially conceived as a “self-service tool set” expanded. Yet, amidst this evolution, we failed to recognize the pivotal juncture where user intuition converges with effectiveness. Encouraged by the growing success and incoming ads-generated revenues, we have failed to check the rear view mirror for the alarmingly growing group of users we were leaving behind. Beginning with lower C2C segments we watched attrition rates starting to climb outside any product’s comfort zone. As our reporting functionalities matured, so did the perceived complexity. With that, the learning curve that our tool set required of sellers got progressively steeper. The efficient generation of actionable insights from incoming data had become more of an art form than an acquired skill. Fewer and fewer sellers had the time available to master it enough for remain profitable.
To be fair to all of us, this was hard to cross-check, as the advertising industry landscape looked rather monochromatic in how it thought of delivering user value. The problem we were starting to see very much resembled the very same one I later encountered later with Facebook ads where the increasing complexity was being remedied by additional reporting functionalities and deep customizations.
This raised some deep questions and forced me to evaluate our approach from a higher altitude.

Sellers struggled to derive insights
Immersive research did not take long to confirm the hypothesis, and revealed evidence of sellers struggling to navigate the depths of incoming metrics and process them into actionable insights. This included swaths of segments whose margins allowed for an initial experiment, but failed to apply those learnings towards a subsequent advertising action. Many deemed the associated risks being too high to justify further allocation of ads budgets and reverted to a more familiar organic means for acceleration of sales.
This raised some deep questions and forced me to evaluate our approach from a higher altitude.

The complex pursuit of simplicity
The unequivocal nature of adoption metrics signaled the emergence of structural faults in our product approach. Yet the momentum of the Ads platform required a sequence of progressively incremental steps in search of a solution. The only sensible course of action would be to methodically address the hypothetical causes, as we narrowed down the list of cost-effective remedies. Subsequently, the effort was made to expand onboarding experience, to introduce inline guidance and real-time guidance along all core user tasks. The goal was to help sellers acquire the required skillsets while using the tools and to develop their own approach to both strategy and tactics. In other words, our solution was to give sellers knowledge on how to deal with the inherent complexity of advertising.
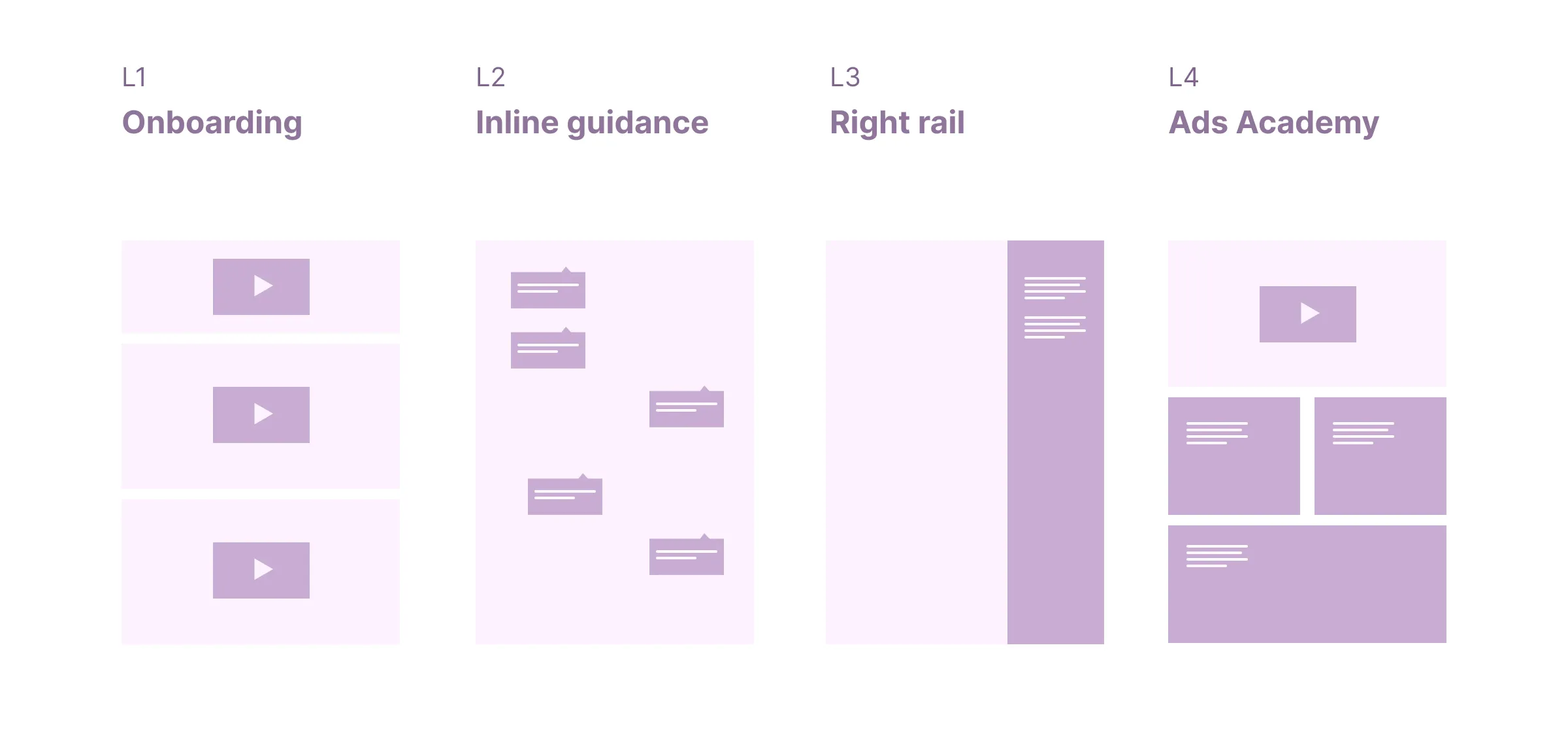
The resulting adoption rates revealed some valuable insights. The middle segments had responded with largest uplift in adoption of Ads tools. We attributed it to their unique business and operational positioning. Simply put, they had the highest motivation to learn along. But these results did little to convince me that the metrics uplift was correlated to the improved overall efficiency. We may have made it more possible to succeed but we had fallen short of making it easier or cost-effective to do so.
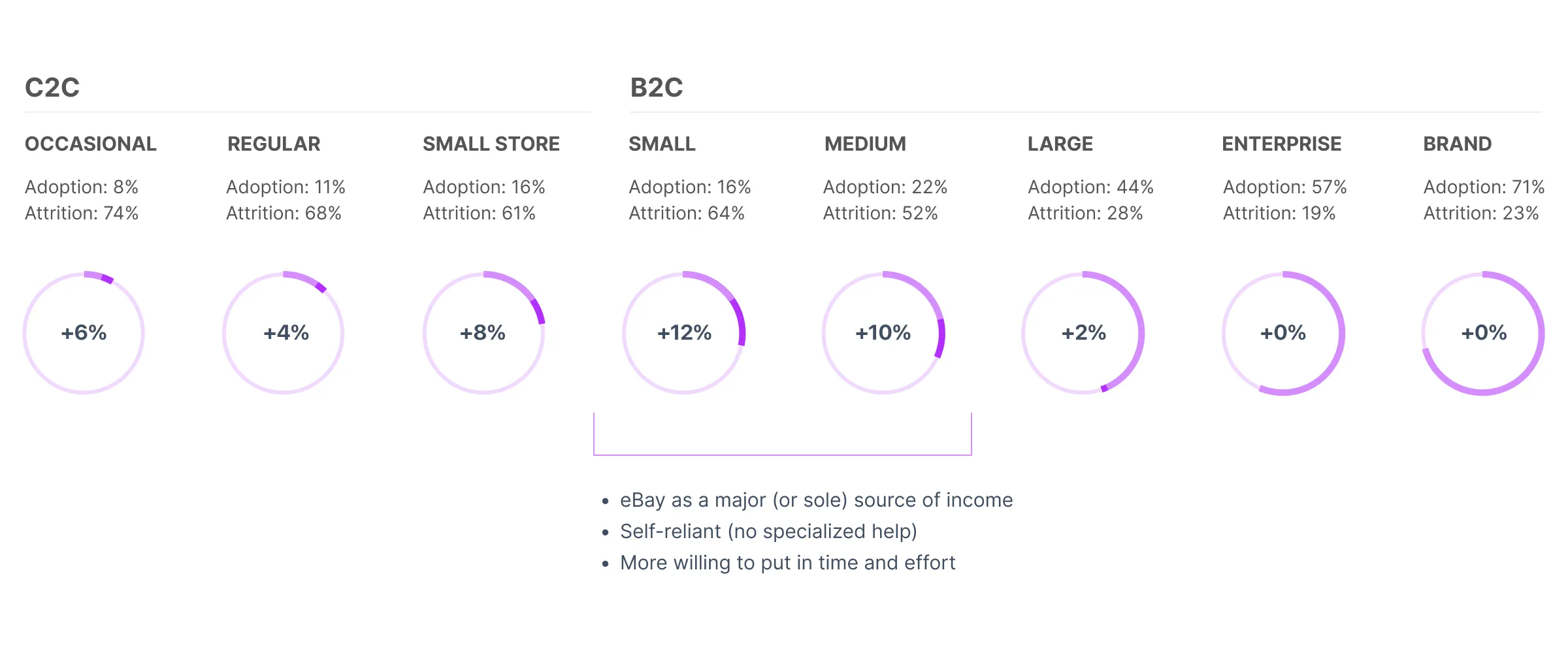
The Law of Conservation of Complexity
A Design System, the interface, and the meticulous distillation of guidance do not serve as a panacea for missing the profound essence of the human element. The human factor will be the ever-present guarantee of friction and confusion within complex frameworks with multiple moving pieces.
The law of conservation of complexity, also known as Tesler's Law, is an adage in human–computer interaction stating that every application has an inherent amount of complexity that cannot be removed or hidden. Instead, it must be dealt with, either in product development or in user interaction.
After the 5-year journey I find myself confronting the ultimate enigma within this grand puzzle: on whose shoulders should the remaining complexity fall? Could it be that the most obvious solution has eluded us all this time?
The path forward

Phase 2: Personalized best actions
By the end of 2021 I had become an advocate for our strategic departure from the “self-service” approach to advertising experience at eBay. The amounted evidence was helping me move the organization’s thinking towards a more targeted and outcome-focused methodology. This was facilitated by the synergy between historical data analysis and the adoption of cutting-edge machine learning technologies, which were keen to undergo real-world testing.
My focus fell on developing a system of personalized recommendations (a.k.a. best actions). Aimed at solving a known point of user friction, they would be contextually into the reporting surfaces they would offer a ready-made solution right at the moment of highest user consideration. They would be tailored by a variety of specific micro-factors, such as inventory type (on item specifics level), current ads saturation per category, and advertising program matching based on projected RoAS.

Outcome-centered recommendations (L1)

Outcome-centered recommendations (L2)

Campaign Dashboard: Outcome-centered recommendations (L2)
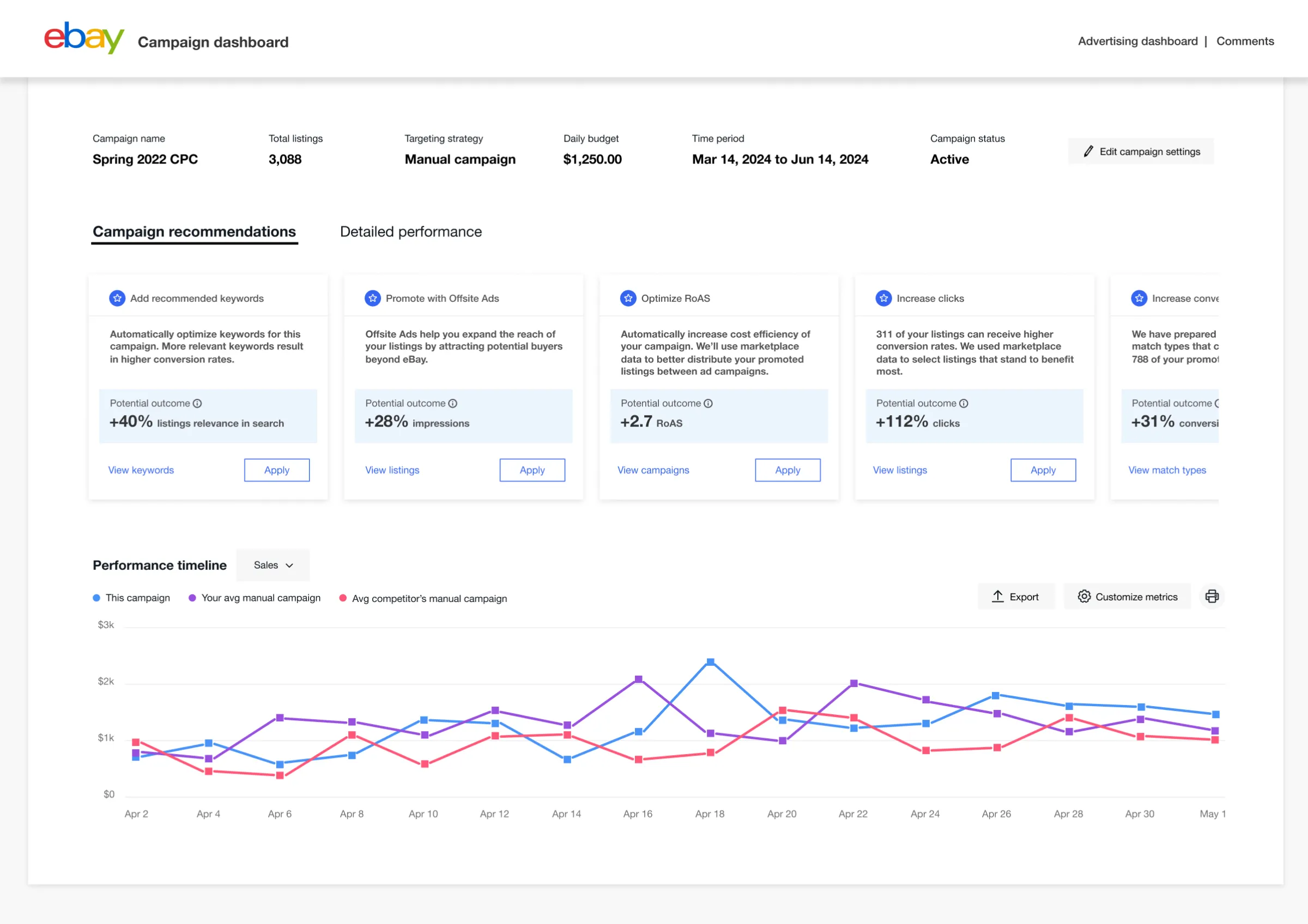
Phase 3: Objective-based advertising
Every time we set foot outside our homes, we do so with an objective in mind. Be that a quick run to the mailbox, a trip across town to a store or an appointment, it begins with a clearly set purpose. This purpose defines the time we allocate, what we wear, what we take along and the route we select for getting there, among variety of other subservient considerations. Advertising works along the same logical outcome-focused string of steps.
Objective-based advertising has become in industry-level norm within last 5 years and has a long evolutionary path ahead. ML-powered (historical data training) takes it a step deeper, where certain complex outcomes are triangulated into likely outcomes by factoring in similar factors in a similar context. As compared to manual campaigns, a much larger pool of data is applied, increasing the likelihood or the outcome and narrowing down the precision of the recommended action. Altogether, this drives advertising profitability while lessening the decision-making burdens on the advertisers. As a result we have reduced friction, higher adoption and the increase in ads-driven sales.
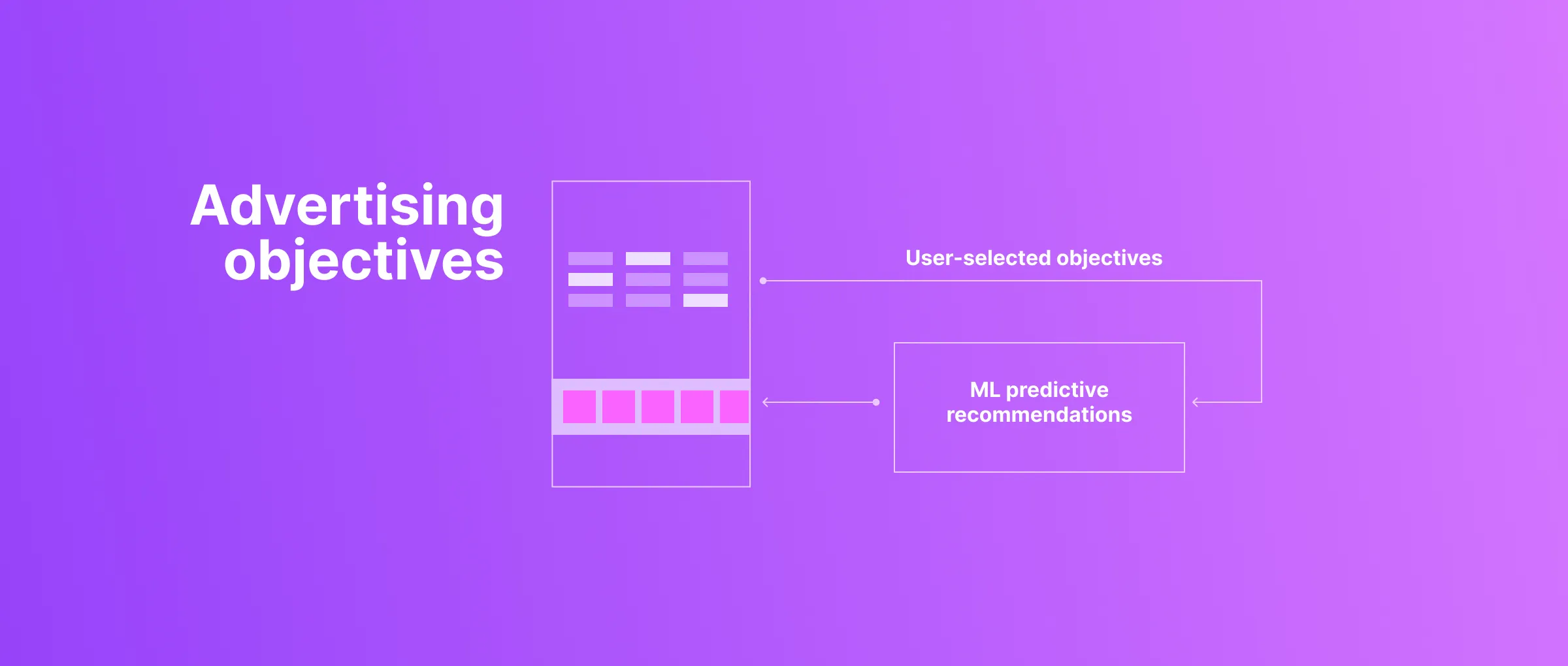
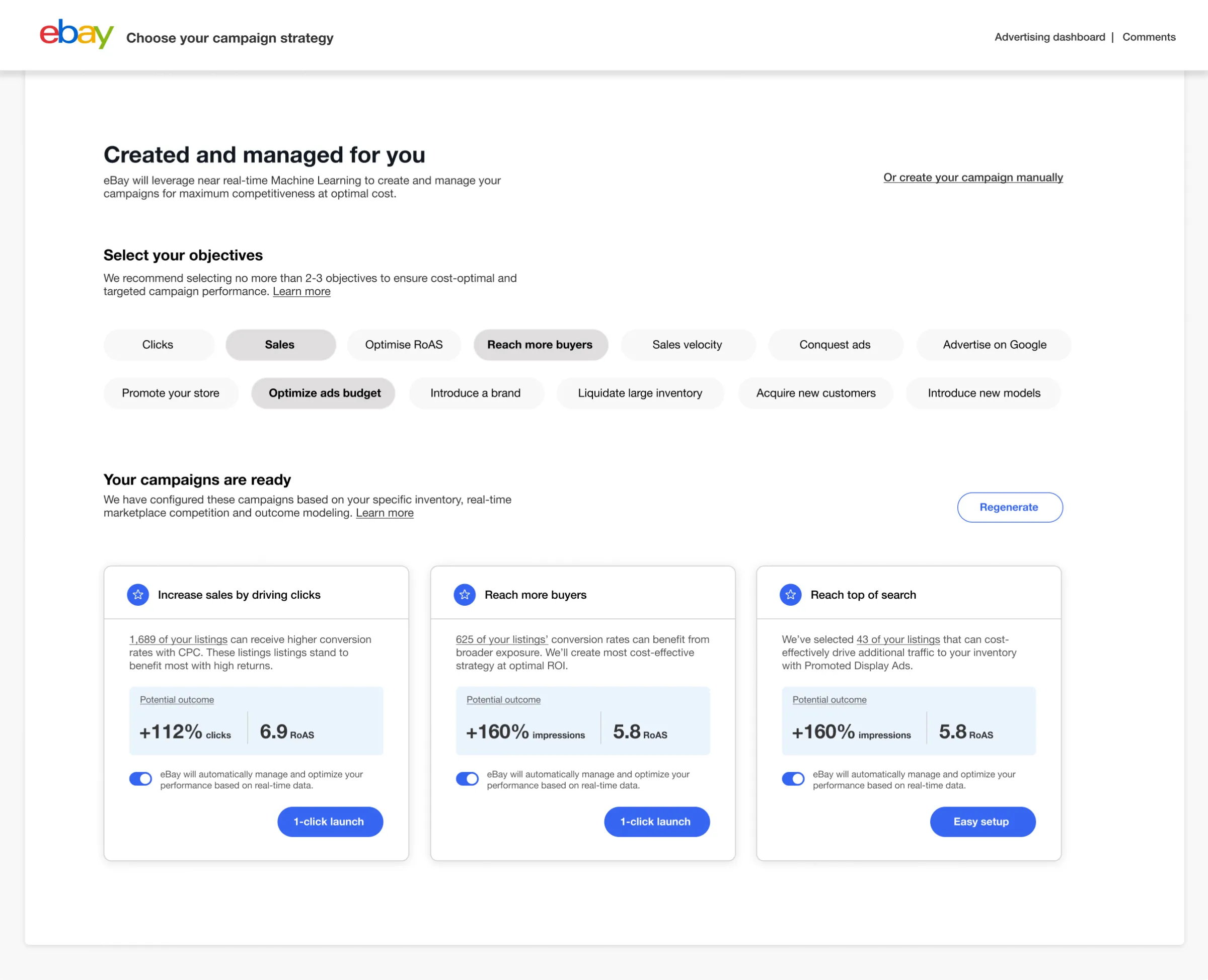
Phase 4: ML-powered advertising
eBay stands at an advantageous crossroads for harnessing the power of machine learning (ML) within its seller and advertising platforms. Unlike numerous companies that scramble to identify tangible AI problems, eBay has amassed a substantial backlog of persistent user challenges—ones that have defied time and our most diligent attempts to eliminate them. It has also amassed a wealth of high-quality comparative data that requires rapid aggregation and its instant translation into best next action. ML provides fast response to these micro-changes and instantly re-tailors selling and advertiser tactics and applies them towards user-defined objective. Furthermore, Large Language Models (LLMs) can deepen the neural-level understanding of the user objectives within the specifics of their inventory and real-time market dynamics.
The rationale behind this lies not in operational intricacies but rather in computational prowess. To thrive in advertising, one must outperform competitors by meticulously allocating ad spend. Decisions such as which program to utilize at a given moment in time, which listings to prioritize, selecting optimal ad rates all extend beyond normal human capacity.
The sheer volume of these variables and their aggregation for instant, precise micro-adjustments can only be delivered by well-trained ML backend.
Key problems that ML can solve


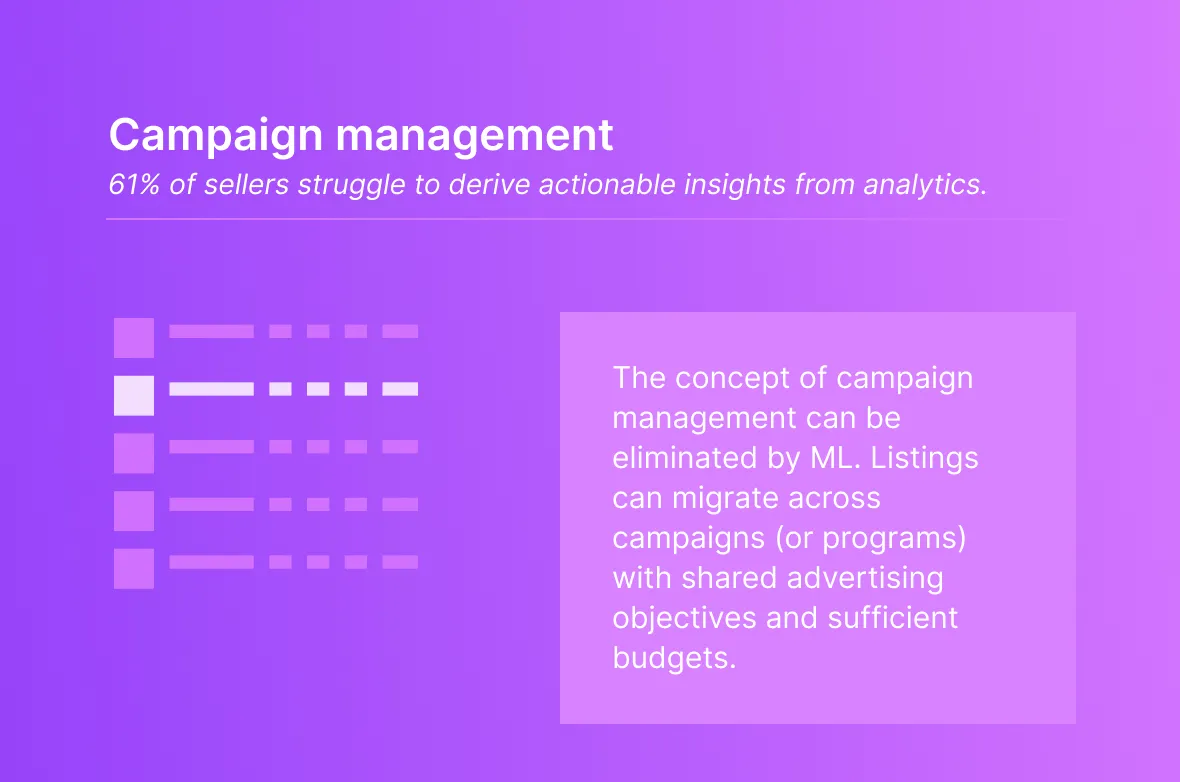
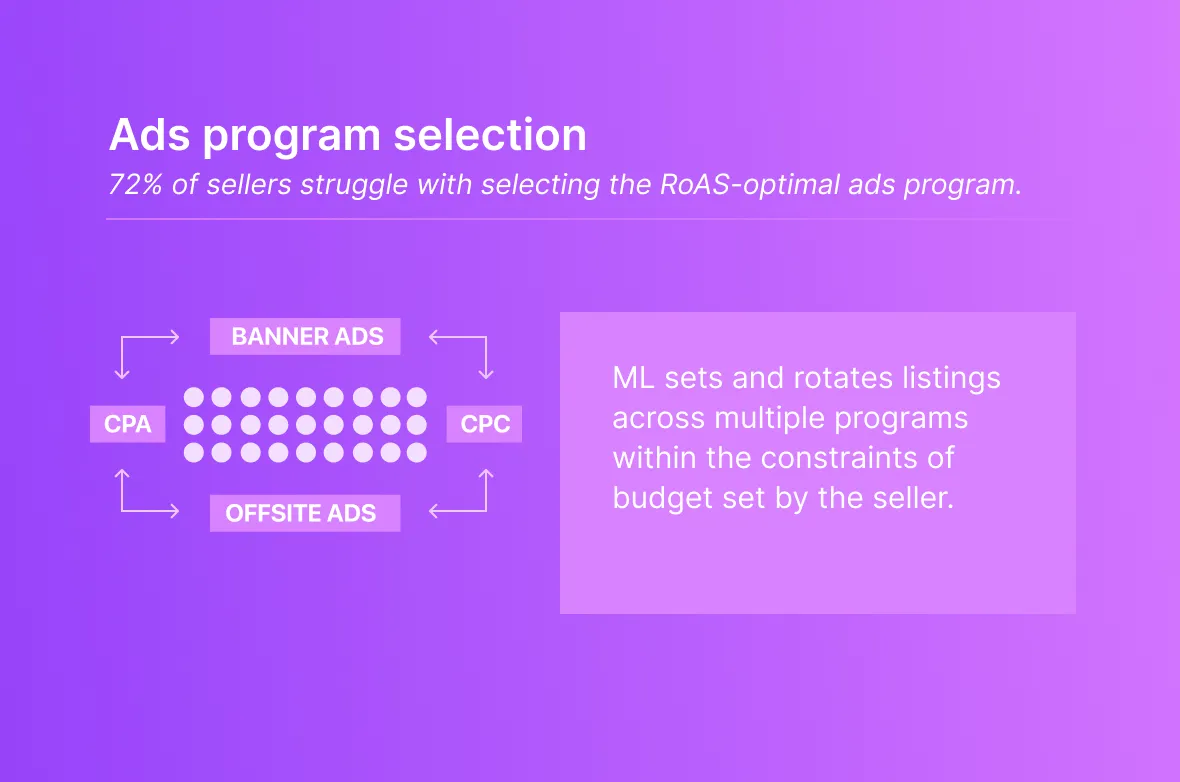
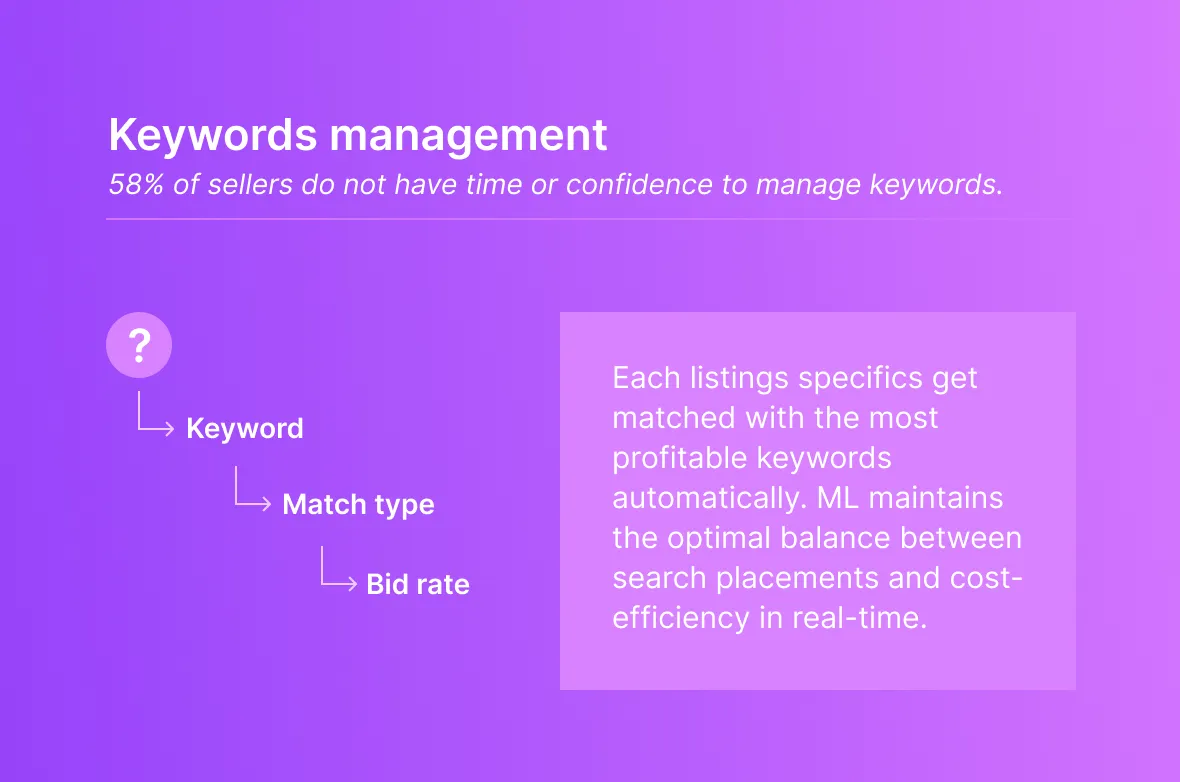
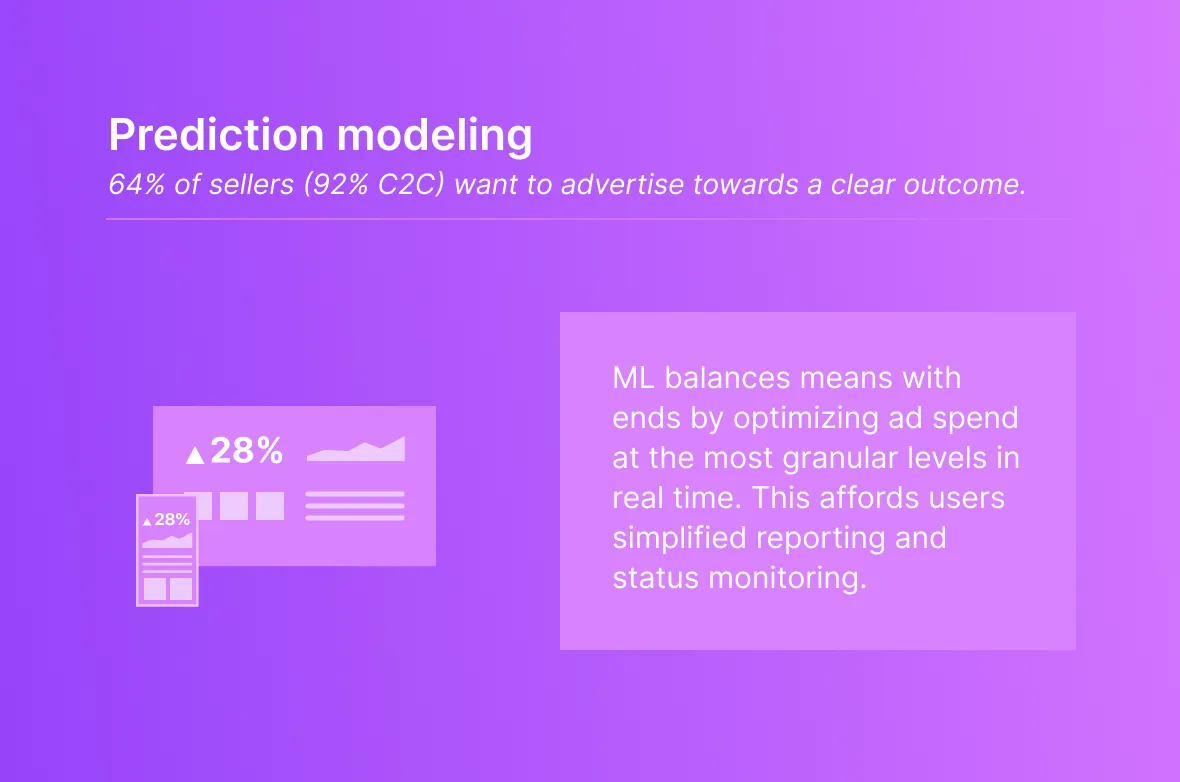
Looking ahead
This journey has placed eBay on a firm vector towards automation of advertising services through Machine Learning. 2022 had marked the intellectual departure from Self-service and put eBay on the road towards creating the full-service automated Advertising platform. Profitable advertising must be symbiotic where the success of users translates directly to the profitability of the platform. Continuous investment in ML capabilities, layered with LLM’s neural sensitivity to user intent will eventually transform advertising at eBay into a fully automated advertising agency.
